|
|
|
project results: |
Learning Probabilistic Structure Graphs
Johannes Hartz
Cognitive Systems Laboratory, Department of Informatics
University of Hamburg
October, 2009
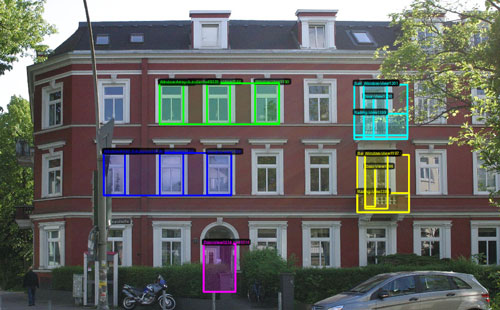 |
|
A novel domain-independent method for learning the probabilistic structure of 2D object configuration has been developed. The approach is graph-based and uses Maximum Common Subgraph Isomorphism to generalise a model graph over a set of training examples. A full probabilistic model, called a Probabilistic Structure Graph (PSG), is obtained by generalising the structural probabilities learnt from the examples. Models learnt from the eTRIMS image database have been used for classifying unknown object configurations, and an average classification rate of ca. 80% has been obtained. Additionally, bottom-up scene interpretation based solely on learnt models has been performed with promising results.
|
References
[1] |
Hartz, J.
Learning Probabilistic Structure Graphs for Classification and Detection of Object Structures
to appear in: Proc. of the of the International Conference on Machine Learning and Applications
2009
[pdf]
|
|
|