Yue Pan
Ph.D. Student Contact:Email: yue.pan@nulligg.uni-bonn.de
Tel: +49 – 228 – 73 – 29 05
Office: Nussallee 15, 1. OG, room 1.003
Address:
University of Bonn
Photogrammetry, IGG
Nussallee 15
53115 Bonn
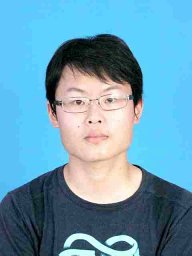
Profiles: Google Scholar | Github | Linkedln
Short CV
Yue Pan is a PhD student at the University of Bonn since June 2022. He received his Master degree in Geomatics in 2022 from ETH Zurich. He received his Bachelor degree in Geomatics Engineering in 2019. He has been a visiting PhD student at ETH Zurich in spring 2024.
Research Interests
- SLAM
- 3D Reconstruction
- Navigation
Projects
- PhenoRob – Robotics and Phenotyping for Sustainable Crop Production (DFG Cluster of Excellence)
Awards
- Geosuisse/IGS prize 2022 for excellent master thesis and academic record in Geomatics
- Robotics: Science and Systems (RSS) Pioneer 2025
Publications
2025
- Y. Pan, X. Zhong, L. Jin, L. Wiesmann, M. Popović, J. Behley, and C. Stachniss, “PINGS: Gaussian Splatting Meets Distance Fields within a Point-Based Implicit Neural Map,” in Proc. of robotics: science and systems (rss), 2025.
[BibTeX] [PDF] [Code] [Video]@inproceedings{pan2025rss, author = {Y. Pan and X. Zhong and L. Jin and L. Wiesmann and M. Popovi\'c and J. Behley and C. Stachniss}, title = {{PINGS: Gaussian Splatting Meets Distance Fields within a Point-Based Implicit Neural Map}}, booktitle= rss, year = 2025, codeurl = {https://github.com/PRBonn/PINGS}, videourl = {https://youtu.be/bbAw_ZC_1fI} }
- X. Zhong, Y. Pan, L. Jin, M. Popović, J. Behley, and C. Stachniss, “Globally Consistent RGB-D SLAM with 2D Gaussian Splatting,” Arxiv preprint, vol. arXiv:2506.00970, 2025.
[BibTeX] [PDF] [Code]@article{zhong2025arxiv, author = {X. Zhong and Y. Pan and L. Jin and M. Popovi\'c and J. Behley and C. Stachniss}, title = {{Globally Consistent RGB-D SLAM with 2D Gaussian Splatting}}, journal = arxiv, year = 2025, volume = {arXiv:2506.00970}, url = {https://www.ipb.uni-bonn.de/pdfs/zhong2025arxiv.pdf}, codeurl = {https://github.com/PRBonn/2DGS-SLAM} }
- L. Jin, X. Zhong, Y. Pan, J. Behley, C. Stachniss, and M. Popović, “ActiveGS: Active Scene Reconstruction Using Gaussian Splatting,” Ieee robotics and automation letters (ra-l), vol. 10, iss. 5, p. 4866–4873, 2025. doi:10.1109/LRA.2025.3555149
[BibTeX] [PDF] [Code]@article{jin2025ral, author = {L. Jin and X. Zhong and Y. Pan and J. Behley and C. Stachniss and M. Popovi\'c}, title = {{ActiveGS: Active Scene Reconstruction Using Gaussian Splatting}}, journal = ral, year = {2025}, volume = {10}, number = {5}, pages = {4866--4873}, issn = {2377-3766}, doi = {10.1109/LRA.2025.3555149}, codeurl = {https://github.com/dmar-bonn/active-gs}, }
- H. Kuang, Y. Pan, X. Zhong, L. Wiesmann, J. Behley, and C. Stachniss, “Improving Indoor Localization Accuracy by Using an Efficient Implicit Neural Map Representation,” in Proc. of the IEEE Intl. Conf. on Robotics & Automation (ICRA), 2025.
[BibTeX] [PDF] [Code]@inproceedings{kuang2025icra, author = {H. Kuang and Y. Pan and X. Zhong and L. Wiesmann and J. Behley and Stachniss, C.}, title = {{Improving Indoor Localization Accuracy by Using an Efficient Implicit Neural Map Representation}}, booktitle = icra, year = {2025}, codeurl = {https://github.com/PRBonn/enm-mcl} }
- F. Magistri, T. Läbe, E. Marks, S. Nagulavancha, Y. Pan, C. Smitt, L. Klingbeil, M. Halstead, H. Kuhlmann, C. McCool, J. Behley, and C. Stachniss, “A Dataset and Benchmark for Shape Completion of Fruits for Agricultural Robotics,” in Proc. of the IEEE Intl. Conf. on Robotics & Automation (ICRA), 2025.
[BibTeX] [PDF] [Code]@inproceedings{magistri2025icra, author = {F. Magistri and T. L\"abe and E. Marks and S. Nagulavancha and Y. Pan and C. Smitt and L. Klingbeil and M. Halstead and H. Kuhlmann and C. McCool and J. Behley and C. Stachniss}, title = {{A Dataset and Benchmark for Shape Completion of Fruits for Agricultural Robotics}}, booktitle = icra, year = 2025, codeurl = {https://www.ipb.uni-bonn.de/data/shape_completion/index.html} }
2024
- Y. Pan, X. Zhong, L. Wiesmann, T. Posewsky, J. Behley, and C. Stachniss, “PIN-SLAM: LiDAR SLAM Using a Point-Based Implicit Neural Representation for Achieving Global Map Consistency,” IEEE Trans. on Robotics (TRO), vol. 40, p. 4045–4064, 2024. doi:10.1109/TRO.2024.3422055
[BibTeX] [PDF] [Code]@article{pan2024tro, author = {Y. Pan and X. Zhong and L. Wiesmann and T. Posewsky and J. Behley and C. Stachniss}, title = {{PIN-SLAM: LiDAR SLAM Using a Point-Based Implicit Neural Representation for Achieving Global Map Consistency}}, journal = tro, year = {2024}, pages = {4045--4064}, volume = {40}, doi = {10.1109/TRO.2024.3422055}, codeurl = {https://github.com/PRBonn/PIN_SLAM} }
- F. Magistri, Y. Pan, J. Bartels, J. Behley, C. Stachniss, and C. Lehnert, “Improving Robotic Fruit Harvesting Within Cluttered Environments Through 3D Shape Completion,” Ieee robotics and automation letters (ra-l), vol. 9, iss. 8, p. 7357–7364, 2024. doi:10.1109/LRA.2024.3421788
[BibTeX] [PDF]@article{magistri2024ral, author = {F. Magistri and Y. Pan and J. Bartels and J. Behley and C. Stachniss and C. Lehnert}, title = {{Improving Robotic Fruit Harvesting Within Cluttered Environments Through 3D Shape Completion}}, journal = ral, volume = {9}, number = {8}, pages = {7357--7364}, year = 2024, doi = {10.1109/LRA.2024.3421788} }
- L. Jin, H. Kuang, Y. Pan, C. Stachniss, and M. Popović, “STAIR: Semantic-Targeted Active Implicit Reconstruction,” in Proc. of the ieee/rsj intl. conf. on intelligent robots and systems (iros), 2024. doi:10.1109/IROS58592.2024.10801401
[BibTeX] [PDF] [Code]@inproceedings{jin2024iros, author = {L. Jin and H. Kuang and Y. Pan and C. Stachniss and M. Popovi\'c}, title = {{STAIR: Semantic-Targeted Active Implicit Reconstruction}}, booktitle = iros, year = 2024, codeurl = {https://github.com/dmar-bonn/stair}, doi = {10.1109/IROS58592.2024.10801401} }
- X. Zhong, Y. Pan, C. Stachniss, and J. Behley, “3D LiDAR Mapping in Dynamic Environments using a 4D Implicit Neural Representation,” in Proc. of the IEEE/CVF Conf. on Computer Vision and Pattern Recognition (CVPR), 2024. doi:10.1109/CVPR52733.2024.01460
[BibTeX] [PDF] [Code] [Video]@inproceedings{zhong2024cvpr, author = {X. Zhong and Y. Pan and C. Stachniss and J. Behley}, title = {{3D LiDAR Mapping in Dynamic Environments using a 4D Implicit Neural Representation}}, booktitle = cvpr, year = 2024, doi = {10.1109/CVPR52733.2024.01460}, codeurl = {https://github.com/PRBonn/4dNDF}, videourl = {https://youtu.be/pRNKRcTkxjs} }
2023
- Y. Pan, F. Magistri, T. Läbe, E. Marks, C. Smitt, C. S. McCool, J. Behley, and C. Stachniss, “Panoptic Mapping with Fruit Completion and Pose Estimation for Horticultural Robots,” in Proc. of the ieee/rsj intl. conf. on intelligent robots and systems (iros), 2023.
[BibTeX] [PDF] [Code] [Video]@inproceedings{pan2023iros, author = {Y. Pan and F. Magistri and T. L\"abe and E. Marks and C. Smitt and C.S. McCool and J. Behley and C. Stachniss}, title = {{Panoptic Mapping with Fruit Completion and Pose Estimation for Horticultural Robots}}, booktitle = iros, year = 2023, codeurl = {https://github.com/PRBonn/HortiMapping}, videourl = {https://youtu.be/fSyHBhskjqA} }
- L. Wiesmann, T. Guadagnino, I. Vizzo, N. Zimmerman, Y. Pan, H. Kuang, J. Behley, and C. Stachniss, “LocNDF: Neural Distance Field Mapping for Robot Localization,” Ieee robotics and automation letters (ra-l), vol. 8, iss. 8, p. 4999–5006, 2023. doi:10.1109/LRA.2023.3291274
[BibTeX] [PDF] [Code] [Video]@article{wiesmann2023ral-icra, author = {L. Wiesmann and T. Guadagnino and I. Vizzo and N. Zimmerman and Y. Pan and H. Kuang and J. Behley and C. Stachniss}, title = {{LocNDF: Neural Distance Field Mapping for Robot Localization}}, journal = ral, volume = {8}, number = {8}, pages = {4999--5006}, year = 2023, url = {https://www.ipb.uni-bonn.de/wp-content/papercite-data/pdf/wiesmann2023ral-icra.pdf}, issn = {2377-3766}, doi = {10.1109/LRA.2023.3291274}, codeurl = {https://github.com/PRBonn/LocNDF}, videourl = {https://youtu.be/-0idH21BpMI} }
- X. Zhong, Y. Pan, J. Behley, and C. Stachniss, “SHINE-Mapping: Large-Scale 3D Mapping Using Sparse Hierarchical Implicit Neural Representations,” in Proc. of the IEEE Intl. Conf. on Robotics & Automation (ICRA), 2023.
[BibTeX] [PDF] [Code] [Video]@inproceedings{zhong2023icra, author = {Zhong, Xingguang and Pan, Yue and Behley, Jens and Stachniss, Cyrill}, title = {{SHINE-Mapping: Large-Scale 3D Mapping Using Sparse Hierarchical Implicit Neural Representations}}, booktitle = icra, year = 2023, codeurl = {https://github.com/PRBonn/SHINE_mapping}, videourl = {https://youtu.be/jRqIupJgQZE} }
2022
- Y. Pan, Y. Kompis, L. Bartolomei, R. Mascaro, C. Stachniss, and M. Chli, “Voxfield: Non-Projective Signed Distance Fields for Online Planning and 3D Reconstruction,” in Proc. of the ieee/rsj intl. conf. on intelligent robots and systems (iros), 2022.
[BibTeX] [PDF] [Code] [Video]@inproceedings{pan2022iros, title = {{Voxfield: Non-Projective Signed Distance Fields for Online Planning and 3D Reconstruction}}, author = {Y. Pan and Y. Kompis and L. Bartolomei and R. Mascaro and C. Stachniss and M. Chli}, booktitle = iros, year = {2022}, codeurl = {https://github.com/VIS4ROB-lab/voxfield}, videourl = {https://youtu.be/JS_yeq-GR4A} }